This is the first of a 2-part blog looking in detail and the evolution of analytics and an example of how it fits into customer success.
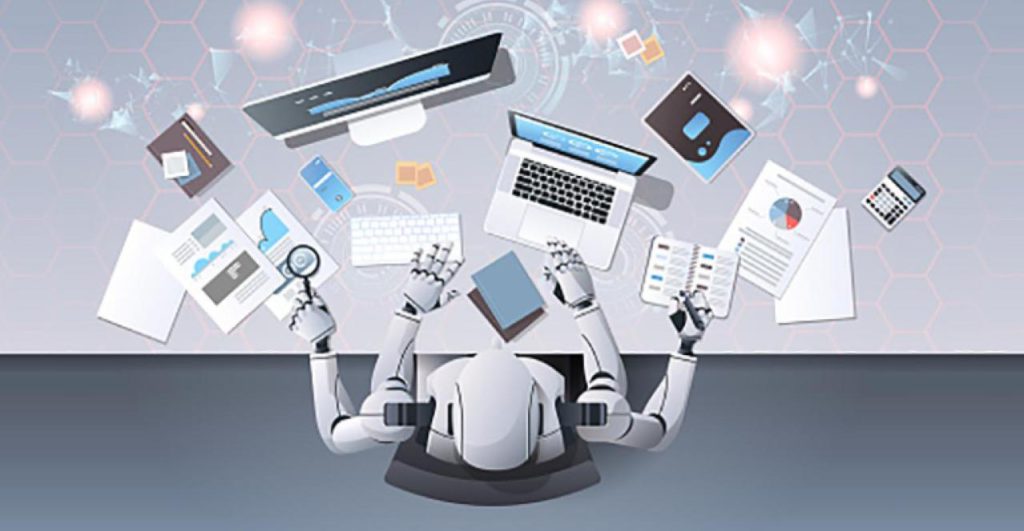
In all or in part, leading organizations are facing revolutionary change from digital transformation. AIOps is altering the nature of IT management. Robotic process automation (RPA) is shifting the way workers do their jobs in fundamental ways.
And AI-driven analytics, such as for marketing activities, sales campaigns, financial reporting, or customer success management, is opening up vastly different and better ways of evaluating and optimizing where and how an organization’s time and dollars are spent.
But “digital transformation” as an approach, especially with regards to “analytics baffles many organizations. For example, just like “the cloud” a decade ago, people understand that “analytics” lead to a better understanding of their business so they can optimize it more effectively.
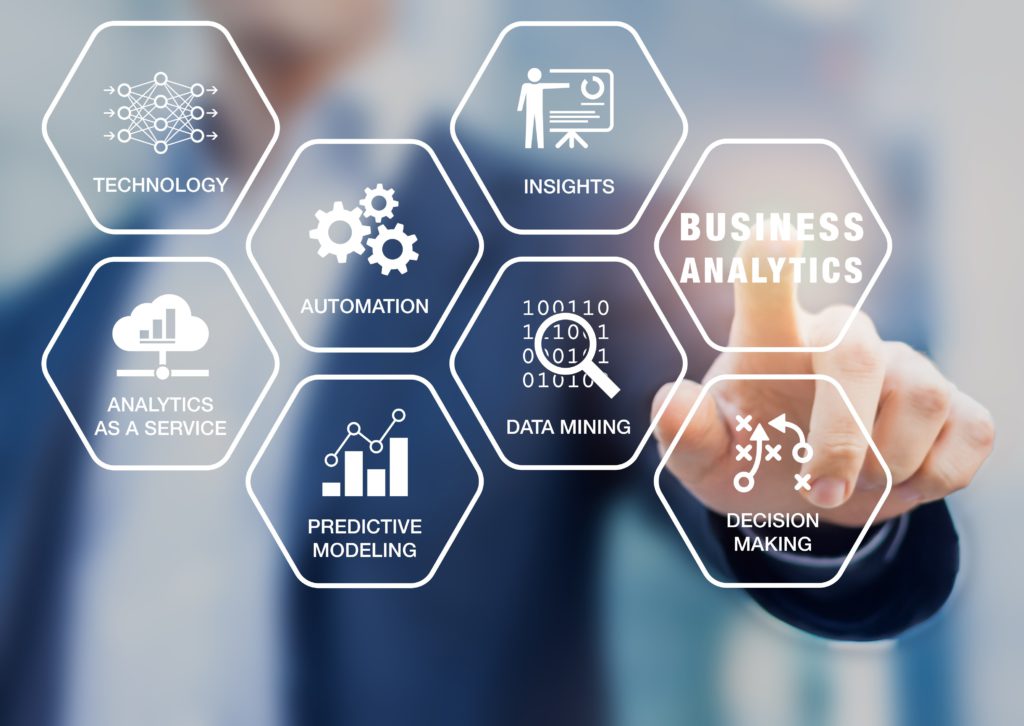
But really knowing what analytics can do, how and where to apply it, how to deploy it in the organization, and how to measure results is unclear at best for most people.
The result is that organizations realize they need to use analytics but have little idea what that means.
Again, like what happened with the cloud, the initial approach to “doing” analytics was for vendors of existing IT platforms to add some limited and focused analytics capabilities to their offerings as an initial step to solve the challenge.
But the limitations of this approach became clear quickly when customers, as they grew to understand better ways to leverage analytics, demanded more sophisticated solutions from these vendor platforms beyond the scope of their architectures.
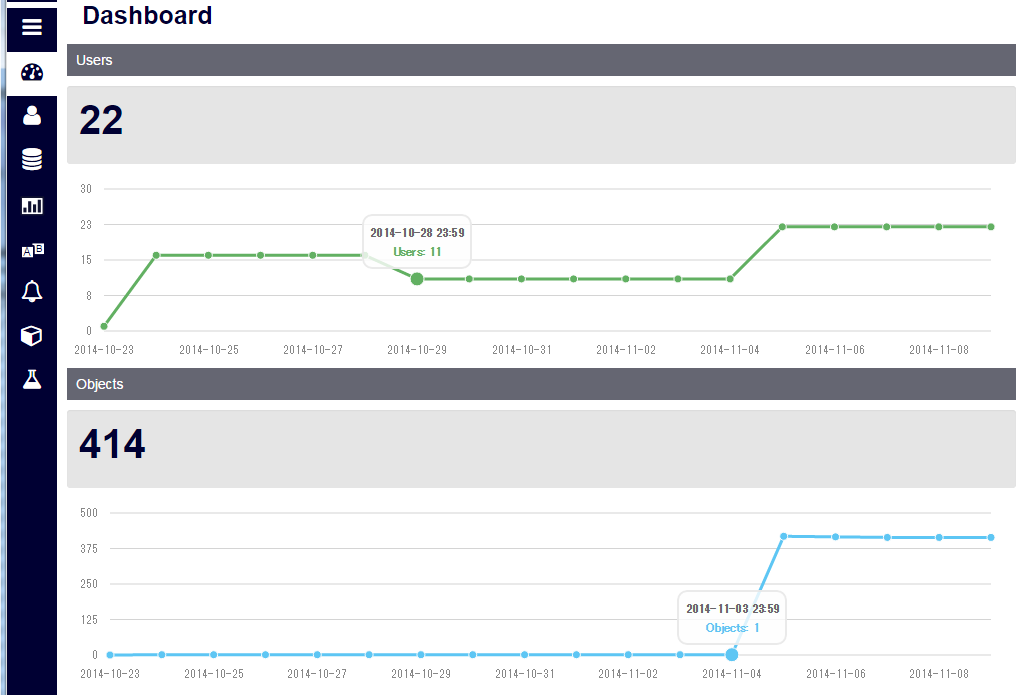
That led to the creation of specialized products and architectures focused on analytics. Initial approaches, while better than bolt-on offerings from legacy application vendors, saw early strong adoption but again proved limited in what those platforms could deliver.
They were mostly dashboards only showing standard statistical metrics that left much of work of understanding and drawing conclusions to the users.
Today, organizations are starting to see solutions incorporating AI and machine learning to help reduce, or even eliminate, the effort users must make to extract conclusions and actions plans from the analytics.

This latest generation is helping organizations realize the full potential of analytics to improve business processes and performance. However, most still require participation of a data scientist to stitch the data and create models in order to generated the most useful insights and predictions.
Example Application: Customer Success
“Analytics” can be applied to a broad variety of business processes and activities. To better understand its potential contribution, let’s look at one specific example: Customer Success.
The focus of customer success is to make sure a customer, once acquired, extracts maximum value from a vendor’s offering while delivering the best possible customer experience throughout their journey with the vendor.
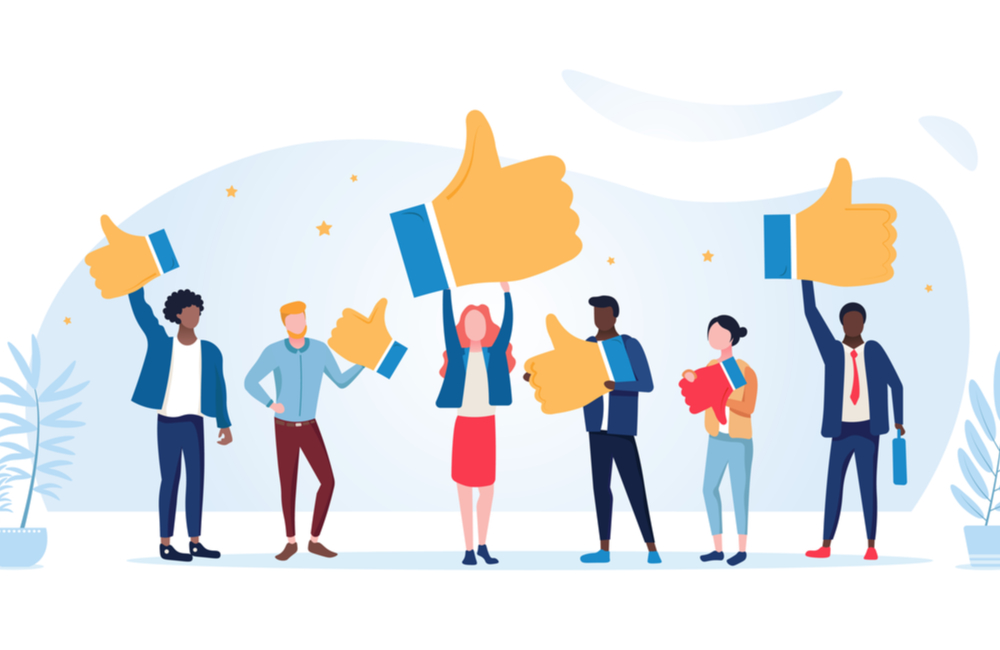
The goal is to convince the customer to continue and expand their commercial relationship. The vendor manages this customer journey using multiple customer touch points to make sure satisfaction remains high.
Historically, these customer touch points happened through individual contact by a vendor rep via phone or digital means. Each rep would manually gather engagement data they thought might be relevant and combine it with their personal experience and knowledge of the customer to create a strategy to grow their business.
But these manual approaches lead to bias and inconsistency in customer management.
In my next blog, I’ll review how technology evolves and map it onto where customer success analytics is heading. I’ll look at each generation of solution and examine the implications of AI and data science.